The Real Deal With Cofounder of Synapse Analytics Galal Elbeshbishy
Guest writer Galal Elbeshbishy shares his insights on how companies can best implement AI whilst minimising its risk.
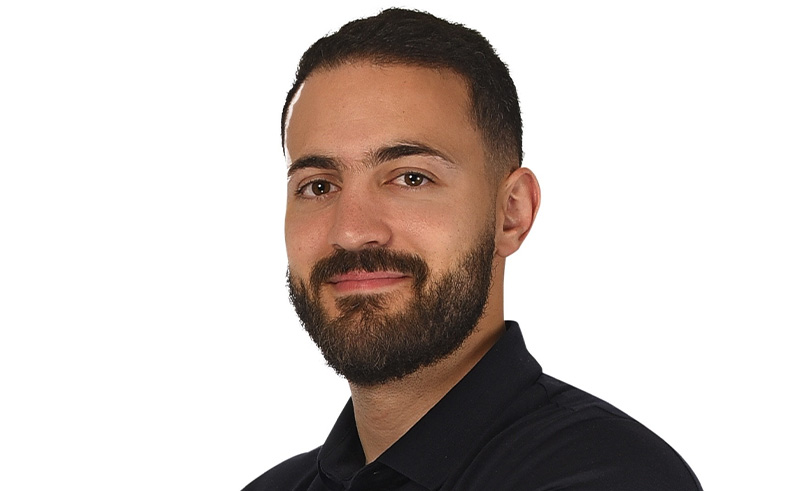
StartupScene’s op-ed series ‘The Real Deal’ asks some of the region’s most dynamic entrepreneurs to share their stories, passions, struggles and personal thoughts on the workings of the ecosystem and their entrepreneurial journeys. This is a glimpse behind the headlines, the raises and the often misleadingly tantalising veneer of the fast-track.
This week our guest writer is Galal Elbeshbishy, COO and Cofounder of Synapse Analytics, an AI and data science company that offers solutions to help organisations better organise and monetize their data. In his in-depth op-ed, Elbeshbishy shares the best ways organisations can introduce AI into their toolkit, and minimise the risk that can come from implementing this exciting yet unpredictable technology.
This is Galal Elbeshbishy’s REAL DEAL…
In 2023 alone, we’ve witnessed an unrelenting hype surrounding the use of artificial intelligence (AI) and emerging technologies. But despite the excitement pertaining to research, academic experimentation, and the wide commercialization of AI chatbots, actually leveraging AI in production is a different story.
In other words, whereas AI can work seamlessly and successfully in the confines of a controlled environment in research facilities, said system proves to be brittle when taken out to the real world. For organizations that want to deploy AI in industrial applications, the task becomes exceedingly difficult. But not impossible.
In what follows, I use my experience in the field to shed some necessary light on the obstacles faced when dealing with AI in industry, and offer some insights on how to sidestep them.
To start with, when considering the adoption of AI in your organization, it can be daunting to determine where to start.
One effective strategy is to identify the right problem to solve with AI and begin with a low touch. Choosing the right problem requires careful evaluation of several factors. This involves identifying a business pain point that has a small number of stakeholders, a concise dataset, a large number of records that is continually growing, low perceived threat to the business stakeholder, and most importantly, measurable impact.
In short, by listing down your business pain points and examining the data that tells the story, you can find the use-case where the cost of getting a prediction wrong is much lower than the benefit of getting a prediction right.
Let’s break this down. First, a low number of stakeholders can simplify the implementation of AI as it requires less coordination among different teams or departments. It can also make it easier to obtain buy-in from those involved in the project.
The type of dataset is instrumental too. A concise dataset with a low to zero continuous need for data cleansing and engineering is ideal. You must ensure that the data is clean, preprocessed, and formatted appropriately for AI use. This means that the data should be relatively easy to clean and not require a significant amount of time or resources to process. Additionally, the dataset should be large and constantly growing, as this ensures that the AI model will improve over time.
It's also essential to select a problem with a low perceived threat to the business stakeholder. Choosing a problem that has a minimal risk associated with it can increase the chances of obtaining buy-in from stakeholders. This, in turn, can make it easier to seamlessly implement AI within the organization.
Finally, what is perhaps the most critical factor to consider when selecting a problem for AI implementation is the potential impact on the business. The problem must have a measurable impact, which means that the impact must be quantifiable in some way. It's important to note that not all AI models need to be highly accurate to have a significant and positive impact on your business. Even moderately accurate models can provide huge benefits, especially if they automate and streamline tasks or help you predict and forecast certain outcomes.
Before building or testing the efficacy of an AI model, it's important to determine whether there is a predictive signal in your data. A predictive signal is the presence of patterns in the data that can be used to make predictions. To determine whether a predictive signal exists, it is necessary to apply your business acumen or expertise to determine whether there exists a reliable predictive signal within your data, before applying AI. A good eye for this can save you significant time and money in selecting the most suitable AI problem for your organization.
Let’s take a step back and take stock of the several factors we’ve considered so far.
As argued earlier, successfully and seamlessly deploying AI models in an organization is considerably challenging. That’s why, for instance, Gartner predicts that AI investments often fail, with a staggering 80% of AI algorithms never moving to production.
This is why it’s essential to carefully consider the ways in which you can increase the chances of successfully implementing AI within your organization. To do so, it is recommended to adopt the above mentioned approach.
To summarize, the approach entails beginning by selecting the right problem to solve with AI, which is critical to its successful implementation within an organization. You should identify a ripe, low touch problem with measurable impact. Afterwards, you should turn your attention towards the following factors: the number of stakeholders involved, the quantity and quality of the dataset, perceived threat to the business stakeholder, and measurable impact. Additionally, it's important to evaluate whether there is a predictive signal in the data and to identify tasks that require human-level comprehension but at scale. Following these steps will help you leverage AI to streamline and optimize your business operations.
- Previous Article Egypt-Based Startups Are Now Exempt from Taxes for Five Years
- Next Article Egyptian Embassies Around the World